In the rapidly evolving world of data, businesses need agile, scalable, and reliable solutions to handle their ever-growing data needs. At Kleene.ai, we are at the forefront of this revolution, leveraging the power of Directed Acyclic Graphs (DAGs) to transform data warehousing.
In this blog post, we’ll explore eight benefits of adopting a DAG-based approach to data modeling and why it’s the best practice for modern SaaS solutions.
1.Flexibility: The Backbone of Modern Data Architectures
Dynamic Orchestration: Unlike traditional linear pipelines, DAGs allow for dynamic orchestration of data transforms and table updates. This means that transforms and tables can be scheduled, managed, and executed based on the current state of the system, providing a highly responsive and adaptable data flow.
Conditional Execution: With DAGs, transforms and table updates can be executed conditionally based on specific criteria or data conditions. This level of control ensures that your data processing is precise and efficient, only running when necessary.
Parallel Processing: DAGs enable the parallel execution of independent transforms, significantly speeding up data processing times. This ability to handle multiple processes concurrently improves overall system performance and ensures timely data availability.
2.Scalability: Grow Without Limits
Distributed Execution: DAGs naturally lend themselves to distributed execution across multiple nodes or servers. This makes it easy to scale your data processing capabilities as your data volumes grow, without compromising on performance.
Resource Optimization: By enabling parallel processing and distributed execution, DAGs ensure optimal utilization of available resources. This not only improves efficiency but also reduces costs associated with underutilized infrastructure.
Fault Tolerance: DAG-based systems are inherently more robust. If a transform fails, it can be retried or recovered without affecting the entire workflow. This fault tolerance is crucial for maintaining reliable data processing pipelines.
3.Maintainability: Keep Your Data Flowing Smoothly
Modular Design: DAGs promote a modular approach to data modeling. Individual transforms can be easily added, removed, or rearranged, making it simpler to update and maintain your data warehouse over time.
Dependency Management: With DAGs, dependencies between transforms and tables are explicitly defined. This clear structure makes it easier to understand and manage the flow of data, reducing the risk of errors and improving overall maintainability.
Reusability: In a DAG-based model, each transform builds upon the results of prior transforms, avoiding duplication of logic and ensuring consistency across data processes. By leveraging the outputs of earlier steps, you create a cohesive and efficient workflow where each transform naturally extends the previous ones. This approach not only saves time but also ensures that your data processing logic remains consistent and streamlined throughout the pipeline.
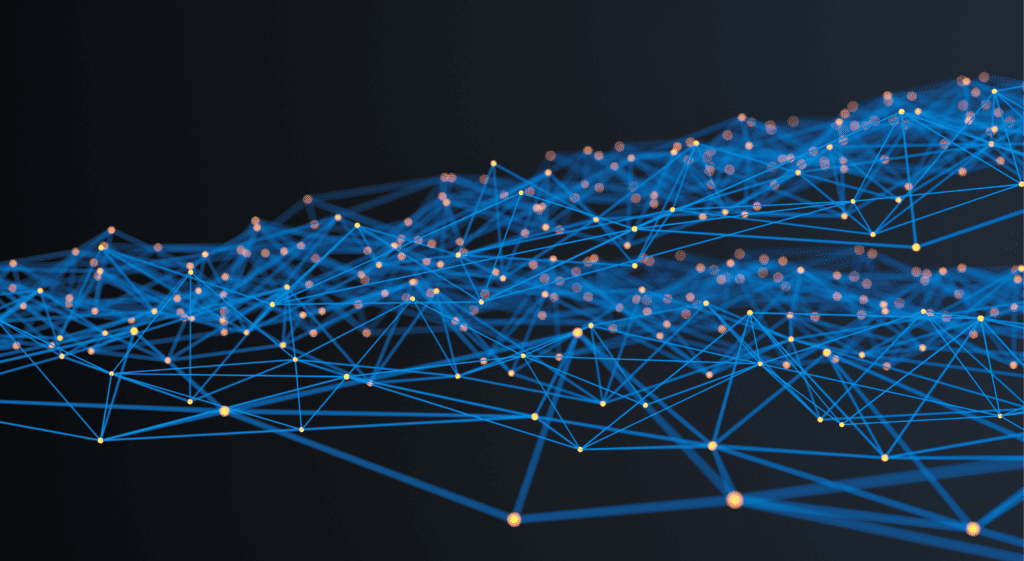
4.Observability: Gain Insights and Control
Lineage Tracking: DAGs inherently capture the lineage of data as it flows through various transformations. This visibility into the data journey is crucial for understanding data dependencies and ensuring data integrity.
Execution Monitoring: Real-time monitoring of DAG-based workflows provides insights into the status and performance of your data processing transforms. This enables proactive management and quick resolution of any issues.
Performance Profiling: DAGs allow for detailed performance profiling of individual transforms. By understanding where bottlenecks occur, you can optimize your data workflows for maximum efficiency.
5.Adaptability: Stay Ahead of Change
Schema Evolution: In a rapidly changing business environment, data schemas often evolve. DAG-based models make it easy to adapt to these changes without disrupting the entire workflow.
Data Source Changes: New data sources can be integrated seamlessly into existing DAGs, ensuring that your data warehouse remains up-to-date with the latest information.
Business Requirement Updates: As business requirements change, DAGs provide the flexibility to update data processing logic quickly and efficiently, keeping your data aligned with business needs.
6.Efficiency: Optimize Every Step
Incremental Updates: DAGs support incremental updates, allowing you to process only the data that has changed. This reduces processing time and improves overall efficiency.
Partial Reprocessing: If errors occur, DAGs enable partial reprocessing of specific transforms rather than rerunning the entire pipeline. This targeted approach saves time and resources.
Optimized Resource Utilization: By leveraging parallel and distributed processing, DAGs ensure that your computing resources are used optimally, reducing waste and lowering costs.
7.Robustness: Build Resilient Data Pipelines
Error Handling: DAGs provide robust error handling mechanisms, ensuring that failures are managed gracefully without impacting the entire workflow.
Retries and Backoffs: Automatic retries and backoff strategies in DAGs enhance the resilience of your data processing, ensuring that transient errors do not derail your data workflows.
Self-Healing Capabilities: Advanced DAG-based systems can incorporate self-healing capabilities, automatically resolving issues and maintaining the health of your data pipelines.
8.Collaboration: Enhance Team Efficiency
Team Coordination: DAGs facilitate better coordination among team members by providing a clear, visual representation of data workflows. This shared understanding improves collaboration and productivity.
Knowledge Sharing: The modular and reusable nature of DAGs promotes knowledge sharing within teams, enabling faster onboarding and cross-functional teamwork.
Cross-Functional Visibility: With DAGs, different teams can gain visibility into the data processes, fostering a collaborative environment where everyone understands how data is being processed and utilized.
Conclusion
At Kleene.ai, we believe that DAG-based data modeling is the future of data warehousing. By offering unparalleled flexibility, scalability, maintainability, observability, adaptability, efficiency, robustness, and collaboration, DAGs are transforming how businesses handle their data. Embrace the power of DAGs with Kleene.ai and unlock the full potential of your data warehouse.
Want to learn more? there are three other ways you can get value from Kleene.ai:
- Download our “A Step-By-Step Guide to Getting From Raw Data to Decision Intelligence” eBook
- Watch our free on demand webinar with Bella & Duke, analysing their growth blueprint and how they optimised their LTV/CAC
- Book a call with an expert and learn how retailers are achieving automated decision intelligence https://kleene.ai/talk-to-an-expert/