By Phil Branston, Former Finance Director Farfetch
It’s been a long and tricky two years for retailers (online and otherwise), with key discretionary markets like fashion clothing finding demand both soft and unpredictable.
Key consumer geographies like the US, the UK, and China have underperformed expectations. As a result, supply chains have become stressed and unpredictable.
Meanwhile, higher interest rates, for more extended periods, have punished excess stock holdings, and fashion brands have had to be tactical in deploying their stock into third-party channels – such as online marketplace – to minimise the damaging effects of discounting as they seek to shift some of this inventory.
Against the backdrop of these significant challenges, because cash is no longer free, equity and debt investors have been demanding an acceleration in the journey towards cash flow break-even. Talk about pressure.
In this article, I will discuss why stock forecasting is back under the microscope — and why AI could be just what the retail industry needs to improve accuracy, efficiency, and profitability.
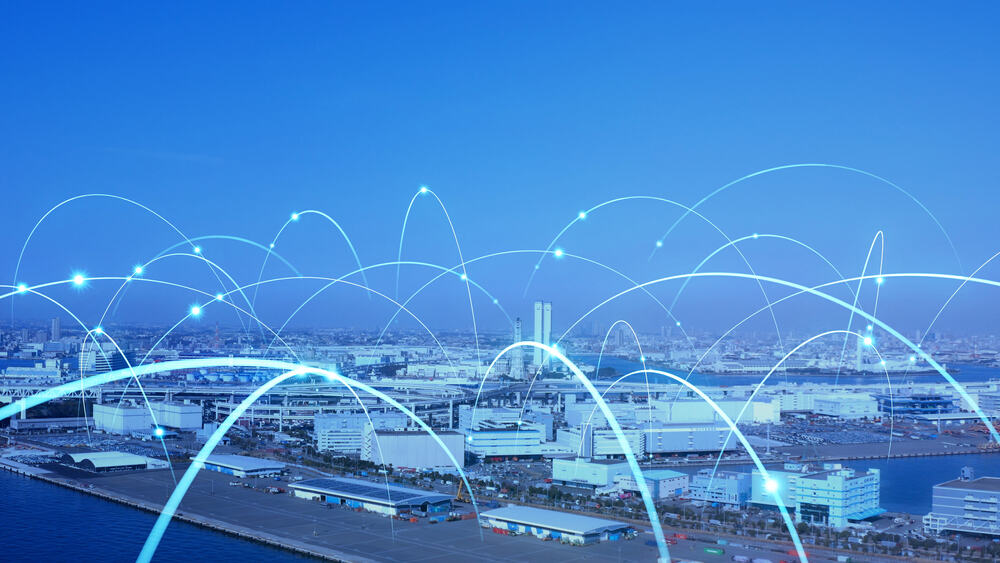
When stock buying became stock piling
In recent years, many online marketplaces (ASOS, Matches, Farfetch) expanded their stock investment (either wholesale from brands or building up their own brands) in an attempt to be less dependent on third-party brand relationships. However, going to into 2023 several found themselves burdened with far too much stock, in a challenging consumer demand environment.
For some, this was uncharted territory. They had to work out the best solution for cash flow quickly, and the choices were stark:
- Keep buying inventory to grow the top line and secure reasonable pricing for new stock in the hope that demand picks up.
- Shrink inventory by any means necessary to raise cash and solidify the balance sheet. But doing this by offering big clearance can reduce gross profits, especially if volumes disappoint, so cash can get hit anyway.
There doesn’t seem to be an elegant way of doing that Goal Seek to optimise cash and profit.
In an ideal world, we would integrate sales forecasting, pricing and promotions, purchase supply intake, and brand partner readiness to supply the new season and old season “continuity” stock.
Unfortunately, it’s not so simple.
Manually managing stock effectively has always been hard
Away from the glamour of new season fashion shows and eye-catching brand collaborations, stock-level management has been an arduous and largely manual task. Re-orders are based on manually reviewing past sales data, but it’s a labour-intensive process that requires a lot of time, resources, and expertise. Even if you have the luxury of that, manual forecasting still lacks the precision of a data-driven solution.
Case in point: Data from McKinsey and Co. shows that companies that have implemented machine learning to handle demand forecasting have achieved 90 per cent accuracy with a three-month lag, compared with around 60 per cent accuracy for manual forecasting methods.
Even today, most retailers are relying on some form of the weekly sales, stock, and intake forecasting model to control and optimise stock levels.
This is driven by supply, with sell-through rates and pricing as external variables. However, the weekly updates can take so long that the WSSI is rarely fully current.
As a result, many online clothing retailers are deploying weekly sales tactics and making potentially vital buying decisions without having an up-to-date WSSI.
The results of this finger-in-the-air manual approach are clear. Keep following this increasingly haphazard path, and you’ll get:
- Poor demand forecasts: Past performance isn’t a guarantee of future outcomes. If you’re building your projections based solely on historical sales data, you won’t have the full picture of what’s driving demand (like marketing activity, promotions, and new product launches). This could lead to you under or overstocking and chasing your tail.
- Lost revenue opportunities: Unnecessary stockouts damage revenue and customer loyalty. If you can’t meet customer expectations at the first ask, they may not give you a second chance.
- Inefficient PO management: When POs are created manually, they drain resources and lengthen replenishment cycles. One bump in the supply chain and you could be looking at lost sales and customer churn.
- Overstocking with no end in sight: Overstocking locks up your capital, increases operating and holding costs, and reduces profit margins. Clothing retailers know this pain all too well when left with an overstock of out-of-season or unfashionable inventory. Other sectors might need to contend with perishables that will expire or spoil. Either way, it’s a frustrating situation that interferes with long-term sustainability.
Why AI inventory management will transform retail
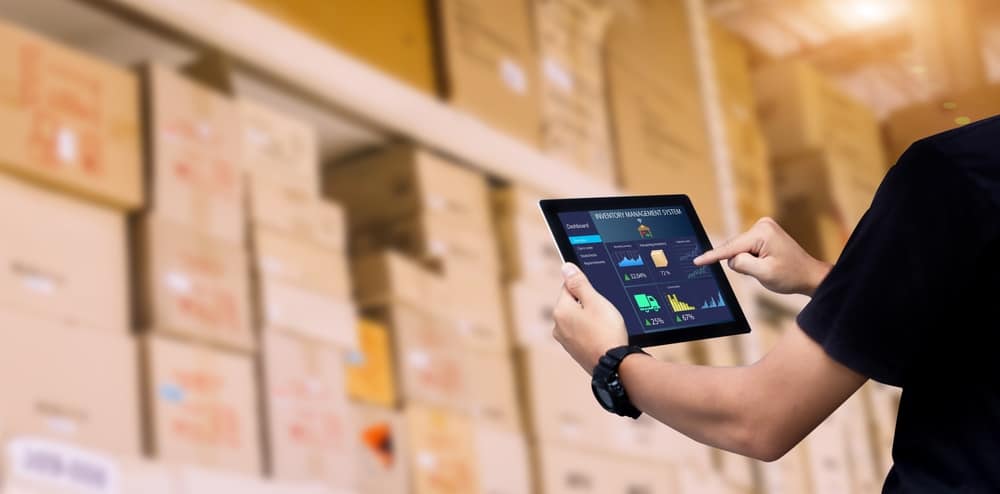
We need to re-establish accurate forecasting as the cornerstone of inventory management, and we can only do that by embracing emerging technologies.
Artificial Intelligence (AI), machine learning, and data analytics offer far more accuracy, agility, and efficiency than manual forecasting methods ever have.
Here’s why:
AI can be leveraged to identify complex underlying patterns that even the sharpest human analysts might miss. By considering scattered insights from historical and real-time marketing and sales data, CRM and ERP systems, economic trends, and even weather patterns, AI can better inform precise stock forecasting for clothing retail brands and marketplaces.
So, how do I see it working? Here are my three main takeaways:
1. AI will help you strategically allocate financial resources
The retail market in general is prone to volatility. Economic fluctuations and industry disruptions (often completely outside our control) can render strategic capital allocation out of step with market reality.
Artificial intelligence can help mitigate against these disturbances to the market and supply chain faster, and help you respond accordingly. When you stop overleveraging by acquiring only the stock necessary to satisfy customer orders, you can free up working capital tied up in aged stock to boost financial agility as you work towards your long-term P&L and cash flow goals.
2. AI will let you forecast demand with unerring accuracy
AI will help you uncover and understand your business’s main demand drivers and improve your stock levels accordingly.
For instance, a platform like Kleene.ai allows you to input data about your future marketing spending, promotions, product exposure, and other factors to build a reliable demand forecast.
In turn, this will streamline inventory management, reduce excess stock, and ensure optimal cash flow (a crucial factor in today’s competitive market).
Avoid stockpiling inventory and free up working capital while reducing wastage and freight costs—the ideal outcome in a rapidly changing market.
3. AI will automate stock management and enhance supplier relationships
Stock management (and especially POs) can be a time-consuming process strewn with human error. An AI platform can ride to the rescue, automatically exporting purchase orders directly into your ERP (or spitting out pre-formatted spreadsheets ready to send to suppliers).
Both outcomes can strengthen relationships with your suppliers, improving supply chain efficiency, reducing lead times, saving money, and boosting service levels.
Summary
Manual inventory management is going out of fashion. Artificial intelligence is à la mode.
By embracing AI, machine learning, predictive analytics and real-time monitoring, retail businesses can gain an accurate view of stock levels and save precious time handling inventory. This leads to proactive decision-making, optimised purchase orders, increased fill rates, and a reduction in costs.
However, implementing AI for inventory and supply chain management requires careful attention to detail. Data quality and scalability are crucial considerations if you’re aiming for growth and sustainability.
A dedicated partner, like Kleene.ai, can help you connect, transform, and visualise your data so that it benefits your bottom line and enhances customer satisfaction through reliable product availability.