Data teams often find themselves stuck in basic data preparation and reporting, struggling to progress to more advanced stages of data utilisation. Decision Intelligence AI represents a significant advancement, empowering data leaders with a potent tool to leverage their data and influence decision-making processes across diverse business functions. This can lead to significant commercial impacts and operational efficiencies. Often this feels practically out of reach to data teams. This blog post aims to delve into the essence of Decision Intelligence AI, outlining its implementation strategies and exploring its transformative applications in crucial business areas, understanding the ways this can go wrong and showcasing a way to achieve the value while avoiding the pitfalls.
Learn about how to climb up the data maturity curve ladder in our free ebook:
https://kleene.ai/e-book/turning-data-into-decisions-in-retail-a-playbook/
What is Decision Intelligence AI?
Decision Intelligence AI combines data science with managerial science to enhance decision-making in business environments. It employs advanced AI technologies, including machine learning and predictive analytics, to interpret vast datasets, predict outcomes, and recommend the best course of action. Unlike traditional analytics, DI AI focuses on generating practical decisions that are directly implementable within a business context, turning data into a strategic asset.
Applications of Decision Intelligence AI
- Marketing: DI AI can revolutionise marketing strategies by predicting customer behaviors, personalising marketing efforts, and optimising budget allocation. For example, by analyzing customer interaction data, companies can tailor marketing messages to individual preferences, significantly increasing engagement and conversion rates.
- Supply Chain: In supply chain management, DI AI provides insights into demand forecasting, inventory management, and logistical optimizations. It enables businesses to anticipate market changes, reduce operational costs, and enhance service delivery. For instance, AI-driven algorithms can predict potential supply disruptions and suggest alternative strategies to maintain business continuity.
- Pricing: DI AI assists in dynamic pricing strategies by analysing market trends, competitor pricing, and consumer demand. This capability enables companies to adjust prices in real-time to maximize revenue and market share. For instance, during high demand periods, prices can be automatically adjusted to reflect the increased consumer willingness to pay, ensuring optimal profitability.
Technical Deep Dive
Decision Intelligence AI harnesses machine learning (ML) models to analyse patterns and predict outcomes from historical data, using algorithms to learn and make informed predictions, such as forecasting sales volumes through supervised learning. Beyond traditional ML, neural networks and deep learning mimic human brain functions to uncover relationships in data, crucial for managing unstructured data like images or speech. Additionally, predictive analytics employs statistical techniques and ML models, such as regression and decision trees, to predict future scenarios essential for tasks like demand forecasting or customer churn. Natural Language Processing (NLP) is also integral, enabling systems to interpret human language, vital for analysing sentiment in customer feedback, automating interactions, or summarising texts for rapid decision-making in Decision Intelligence systems.
4 pillars for successful AI implementation
For Decision Intelligence (DI) AI to excel, several key prerequisites must be met.
- The foundation of trust in DI AI systems is built on data quality, ensuring that the data is accurate, comprehensive, and representative of varying conditions (depth and breadth).
- The system must be tailored to the specific nuances of your business, ensuring that all models and insights are relevant to your unique operational context.
- Practical applicability is crucial; the system should not only provide theoretical insights but also actionable recommendations that can be consumed easily by commercial teams and implemented effectively in real-world scenarios.
- Lastly, flexibility is essential, as the system needs to be robust enough to adapt to unexpected events and changes in the environment, allowing businesses to remain agile and responsive.
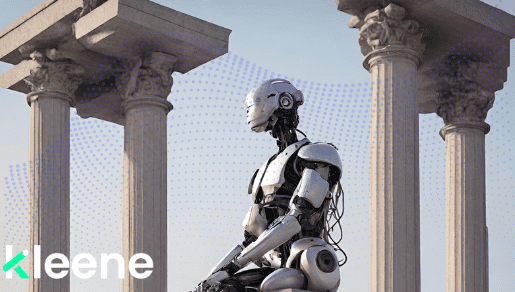
These prerequisites ensure that DI AI systems are not only intelligent but also integral and adaptable to the dynamic business landscape.
The skills gap – Why data teams struggle to deliver this
To effectively harness the power of data analytics and machine learning, by building from scratch, a diverse set of skills is essential.
First, data ingestion skills involve understanding various data formats and the use of tools to import data from multiple sources accurately. Following ingestion, data cleaning is crucial, requiring attention to detail to detect inaccuracies or inconsistencies in the data and skills in using software to clean and organize large datasets efficiently.
Data modeling capabilities are necessary to construct models that accurately represent the underlying structure of the data, which supports predictive analytics and other data science tasks. Analyzing data with commercial specialist knowledge means combining analytical skills with industry-specific insights to derive meaningful conclusions that drive business decisions. To apply machine learning, one must have a strong foundation in algorithms and statistics, along with programming skills to build, train, and deploy models.
Finally, creating usable data applications demands proficiency in software development and user interface design, ensuring the data-driven applications are accessible and beneficial for end-users. Together, these skills form the backbone of a robust data analytics strategy that can transform raw data into actionable insights and real-world solutions.
This represents a huge team overhead for many organisations which is out of reach. Leveraging solutions which build on the work a typical data team is doing and can unlock advanced DI capabilities is key to making the leap to driving that business impact.
The flexibility gap – why point solutions or marquee projects fail
Point solutions or high-profile projects often fail in the realm of Decision Intelligence (DI) AI as, by their nature, they are typically designed to address specific, isolated issues without consideration for the broader operational context or future changes. These solutions may lack the comprehensive data quality necessary to build trust or fail to align with the unique nuances of a business’s operations. Moreover, while marquee projects may impress stakeholders with their scale or ambition, they often falter in practical application, providing theoretical rather than actionable insights. Crucially, both point solutions and marquee projects frequently suffer from a lack of flexibility—they are not built to adapt to unexpected changes in the business environment, leaving organizations rigid in dynamic markets. The absence of these core elements—particularly flexibility—can lead to the underperformance of DI AI initiatives, underscoring the necessity for adaptable, integrated solutions that are responsive to the ever-evolving business landscape.
Bringing this together to create a practical solution
To effectively implement Decision Intelligence AI, it’s important to leverage the existing infrastructure and capabilities of data teams. Data teams typically have a strong grasp of operational contexts and the dynamic nature of businesses, making data warehouses an ideal foundation for AI. The systems already in place for maintaining these data warehouses serve as a natural starting point for building out AI capabilities.
The business intelligence (BI) layer addresses issues related to configuration and operational usability, which are also supported by the data team. This BI layer facilitates the integration of AI by providing a user-friendly interface for interacting with complex data systems.
However, there are gaps in application UX, machine learning, and domain-specific AI knowledge. These gaps can be bridged by incorporating off-the-shelf machine learning and data application solutions. These solutions can be configured to adapt based on the data input provided by data teams, thereby enhancing the overall functionality and effectiveness of AI systems. By utilising these readily available technologies, organisations can accelerate the deployment of AI without the need for extensive custom development
Conclusion
As data leaders, embracing Decision Intelligence AI can significantly uplift your strategic capabilities, turning complex data into actionable and impactful business decisions. Whether optimizing your marketing strategies, streamlining your supply chain, or enhancing pricing models, DI AI stands as a pivotal tool in the modern business arsenal. Building from scratch can be costly and time consuming. Leveraging central data stores and existing skills and deploying solutions designed to bridge the last mile of ML and user consumability can significantly accelerate the journey.
Want to learn more? there are three other ways you can get value from Kleene.ai:
- Download our “A Step-By-Step Guide to Getting From Raw Data to Decision Intelligence” eBook
- Watch our free on demand webinar with Bella & Duke, analysing their growth blueprint and how they optimised their LTV/CAC
- Book a call with an expert and learn how retailers are achieving automated decision intelligence https://kleene.ai/talk-to-an-expert/