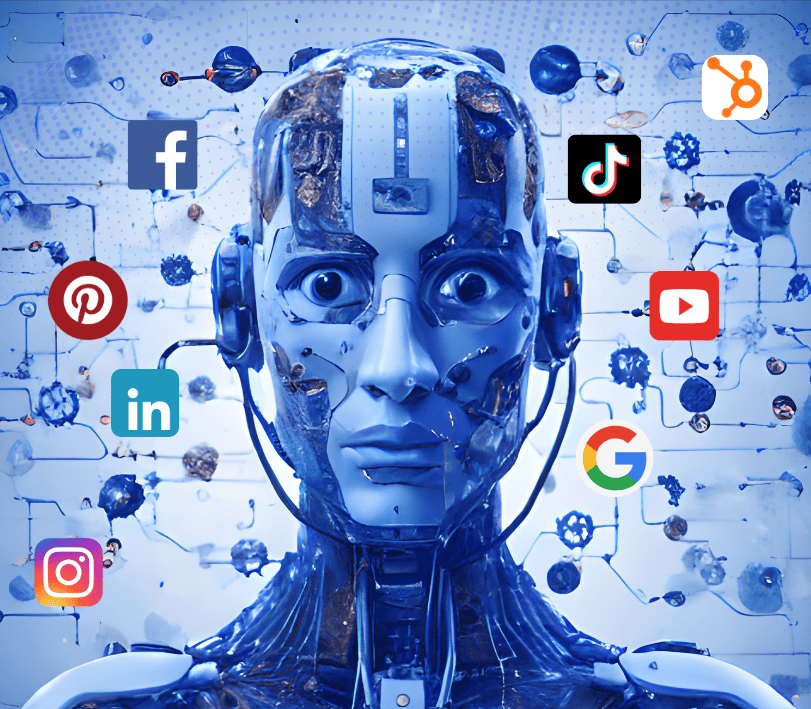
Marketing leaders are often entangled in a web of disparate data sources, struggling with siloed systems that scatter vital information across the organisation. The pursuit of cohesive, actionable insights is hampered not only by the need for extensive data cleansing and integration—resolving user identities and contextualising ad channels—but also by the requirement to conduct complex analyses on large data sets. Moreover, for these insights to be truly transformative, they must be presented through intuitive user interfaces and be deeply informed by an understanding of domain-specific challenges.
Effective decision-making demands that analyses not only be clear and comprehensible but also flexible enough to adapt to evolving business conditions and customer preferences. This blog post delves into the world of Decision Intelligence AI (DI AI), exploring how it equips CMOs to surmount these challenges and harness their data for a competitive edge.
In this blog we’ll discuss:
- What is decision intelligence AI and how it can be applied to marketing
- Implementation strategies and pivotal marketing applications
- Common pitfalls and how to address them
- Outline how marketing teams can fully leverage AI to enhance decision-making and drive better marketing performance.
But first lets’ start with a statistic:
What is Decision Intelligence AI?
Decision Intelligence AI represents a transformative leap in how marketing leaders approach data and decision-making. This cutting-edge field merges data science with managerial science, aiming to elevate the process of marketing decision-making from mere data analysis to strategic action. At its core, AI employs advanced artificial intelligence technologies such as machine learning and predictive analytics. These technologies are capable of sifting through vast amounts of marketing data, predicting outcomes, and prescribing actionable steps that directly align with strategic marketing objectives.
Unlike traditional analytics, which often focus on descriptive and diagnostic analyses, DI AI is proactive and prescriptive. It doesn’t just tell you what has happened or why; it advises on what should happen next. This proactive approach is pivotal for marketing leaders as it converts raw data into a strategic asset, making it not only informative but also operationally transformative. By integrating insights directly into marketing strategies, DI AI turns complex data sets into clear, actionable recommendations that drive tangible business outcomes. It elevates teams into fast moving, data informed strategic operators.
Applications of Decision Intelligence AI
Decision Intelligence AI is instrumental in refining several key areas of marketing, each essential to enhancing overall marketing effectiveness and efficiency. By harnessing DI AI, marketing leaders can optimise their strategies and operations across various dimensions:
- Marketing Spend Attribution: One of the primary applications of DI AI is in elucidating the true return on investment (ROI) for each marketing channel, tactic, and campaign. Traditional methods often struggle to accurately track and attribute the impact of complex customer journeys that involve multiple touchpoints. DI AI steps in to untangle these journeys, identifying which interactions actually drive value. This insight allows marketing leaders to eliminate ineffective spending and reallocate budgets towards the strategies that have proven their worth, ensuring every dollar spent is optimised for maximum impact.
- LTV and Churn Analysis: Understanding customer lifetime value (LTV) and identifying potential churn risks are critical for sustaining long-term business growth. DI AI excels in predicting these metrics, offering marketers the foresight needed to implement timely interventions. By recognizing which customers are likely to churn, and understanding the factors contributing to their potential departure, DI AI enables targeted strategies to nurture high-value customers and reduce turnover, thereby protecting and potentially increasing revenue streams.
- Campaign Optimization: Effective campaigns are not just creatively appealing but are also perfectly timed and targeted. DI AI enhances campaign performance by analysing detailed customer behaviour data. This analysis helps in optimising targeting strategies, tailoring messaging, and choosing the optimal timing for engagement. The result is higher engagement rates, improved conversion rates, and an overall better customer experience, as campaigns resonate more deeply with the intended audience.
- Data-driven Content Strategy: In the content-heavy landscape of modern marketing, making your voice heard above the noise is a significant challenge. DI AI aids in crafting a compelling content strategy by analysing trends, audience preferences, and content performance not just across your own channels, but also competitively. These insights ensure that the content created is not only relevant and engaging to the target audience but also outperforms competing messages in visibility and impact.
The Skills Gap – Why Marketing Teams May Struggle
While marketing teams are typically well-equipped with indispensable business acumen and a profound understanding of customer needs, fully leveraging the capabilities of Decision Intelligence AI often demands specialised expertise that goes beyond traditional marketing skills.
Here are some critical areas where gaps might appear:
- Data Engineering: Effective AI deployment relies on the ability to collect, cleanse, and transform raw data from diverse sources like website analytics, social media, CRM systems, and more. Marketers often lack experience in handling large, complex datasets, along with the necessary tools for rigorous data preparation. This gap can hinder the ability to fully utilise DI AI, as clean and well-structured data is fundamental to accurate analysis.
- Statistical Analysis and Machine Learning Expertise: Properly leveraging DI AI requires not only interpreting AI model outputs but also building and fine-tuning these models. A solid grounding in statistical concepts, such as hypothesis testing, confidence intervals, and model evaluation, is crucial for distinguishing robust insights from mere correlations. Additionally, familiarity with various machine learning algorithms (e.g., decision trees, neural networks), feature engineering techniques, and performance metrics enables marketers to optimise models for specific marketing use cases. Without these combined skills, marketing teams might struggle to effectively harness DI AI outputs in a way that is both relevant and impactful.
- Domain-Specific AI Challenges: Applying AI in marketing involves navigating the intricacies of operational marketing tasks that require specialised knowledge. For example, effective Google ad bidding demands a deep understanding of auction dynamics and keyword optimization, which DI AI can streamline if properly programmed with these operational insights. Additionally, the increasing restrictions on tracking technologies, such as cookie blocking, pose significant challenges in customer tracking and data collection. This impacts the ability to perform accurate marketing spend attribution and understand customer behaviours across different touchpoints. Practical deployment of DI AI also requires solutions to accurately measure spend effectiveness in these constrained environments. Thus, the adoption of DI AI in marketing not only calls for technical AI expertise but also a profound grasp of day-to-day marketing operations and regulatory challenges, ensuring that solutions are both effective and compliant with evolving digital marketing standards.
- UX and Usability: Beyond the analytical capabilities, the effectiveness of DI AI also depends significantly on user experience and usability. Tools and platforms must be designed with the end-user in mind, providing interfaces that are intuitive and facilitate easy access to complex functionalities. Without a strong emphasis on UX, even the most sophisticated DI AI systems can become underutilised, as marketing teams may find them too cumbersome or unintuitive to integrate into their daily operations.
While modern marketing teams include smart individuals with data skills, the manual nature of data manipulation and the scale and complexity of the required analyses often bog these teams down. This creates a bottleneck where the potential of DI AI is recognized but not fully realised.
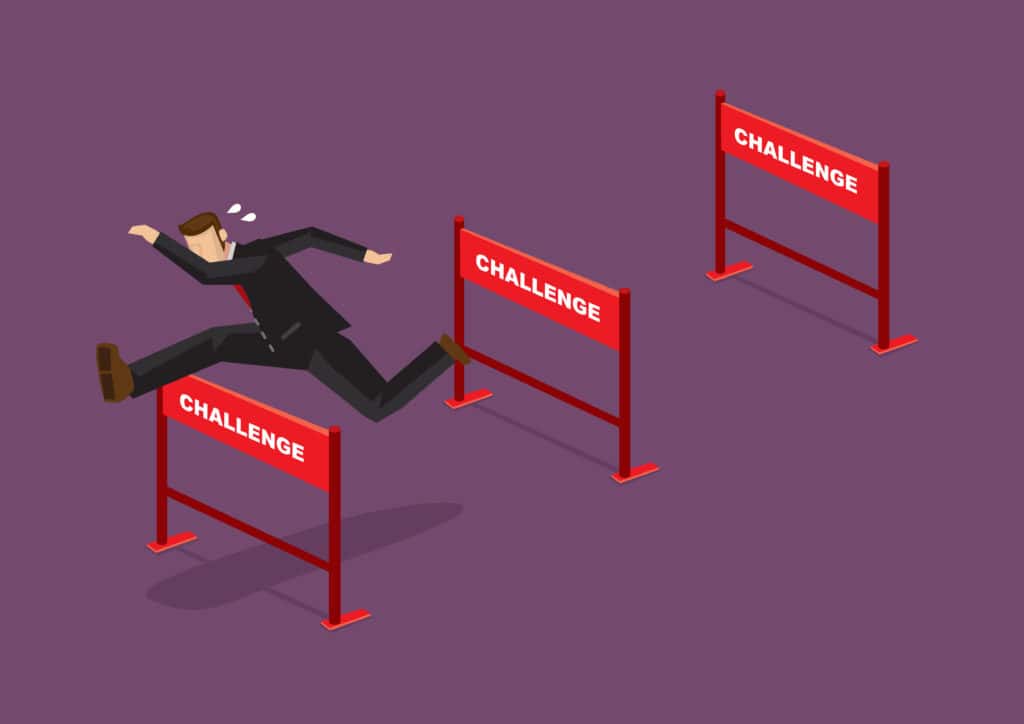
The Flexibility Gap – Why Standalone Tools Fail
Standalone marketing technology tools and ambitious in-house projects frequently encounter significant limitations when it comes to delivering comprehensive Decision Intelligence AI functionality. These limitations can prevent marketing teams from fully capitalising on the potential of AI, ultimately impacting the efficiency and effectiveness of their marketing efforts.
- Limited Data Integration Capabilities: Many tools on the market are not built with the flexibility to handle diverse data sources, which is a fundamental requirement for effective DI AI. When tools fail to integrate data seamlessly from various channels—such as social media platforms, CRM systems, and offline data sources—marketing teams struggle to develop a unified view of the customer journey. This limitation hinders the ability to generate accurate, actionable insights that are crucial for strategic decision-making.
- Misalignment with Marketing Strategy: Standalone tools often offer generic solutions that are not tailored to the specific strategic needs of a business. Without the ability to customise features and functions to align with an organisation’s unique marketing strategies, these tools become less relevant and can even lead to misguided decisions that do not support the overall business objectives.
- Difficulty Adapting to New Data Sources and Marketing Initiatives: The marketing landscape is continuously evolving, with new data sources emerging and marketing tactics being developed. Standalone tools frequently struggle to adapt to these changes promptly. This inflexibility can leave marketing teams unable to leverage new opportunities or insights that could be captured from emerging data streams or innovative marketing techniques.
- Complexity and Lack of Customisability: The integration tools often provided with standalone systems are limited in their functionality and lack the customizability needed to tailor processes to specific organisational needs. This can make working with data at scale particularly challenging and often requires the use of multiple tools to meet all the needs of a marketing department.
- Resource Intensiveness: Utilising multiple tools to cover all aspects of marketing data analysis and decision-making inevitably leads to significant resource consumption. Each tool may require separate maintenance, updates, and training, all of which consume time and financial resources. This fragmentation can lead to inefficiencies and increased costs, detracting from the strategic focus and agility of the marketing team.
Bringing This Together to Create a Practical Solution
For Chief Marketing Officers (CMOs) aiming to fully exploit the capabilities of Decision Intelligence AI (DI AI), the path forward involves constructing a framework that not only bridges the existing skills and technology gaps but also amplifies the strengths of the marketing team. This approach ensures that DI AI is not just a tool, but a core component of the marketing strategy that drives measurable commercial impact.
1. Focusing on Commercial Impact: The primary goal for CMOs should be to leverage DI AI in ways that directly contribute to the business’s bottom line. This means deploying DI AI solutions that enhance decision-making in critical areas such as marketing spend attribution, customer lifetime value (LTV) analysis, and churn prediction. By focusing on these areas, DI AI can directly influence revenue optimization and cost efficiency.
2. Empowering Existing Teams: Any DI AI solution should be an enabler for the existing marketing team, not a replacement. This involves choosing technologies that complement the team’s skills and enhance their capabilities without overwhelming them with complexity. Solutions should integrate seamlessly into current workflows, augmenting the team’s efforts with advanced analytics and deeper insights.
3. Seamless Integration with Current Systems: The effectiveness of a DI AI solution is significantly enhanced by its ability to integrate effortlessly with existing data systems. This integration enables a unified view of data from various sources, providing a comprehensive dataset for analysis. Seamless integration reduces the operational friction and learning curve associated with adopting new technologies.
4. Utilising Advanced Machine Learning and AI: Specialized DI AI solutions apply sophisticated ML and AI technologies to carry out complex tasks such as spend attribution and churn analysis. These technologies can automate the heavy lifting of data processing and insight generation, allowing marketing teams to focus on strategy and execution rather than data management.
5. User-Friendly Interfaces for Marketers: To truly make DI AI accessible and actionable, the solutions must provide user-friendly interfaces that allow marketers to easily understand and act upon the insights generated. These interfaces should be designed with the end-user in mind, ensuring they are intuitive and provide actionable information at a glance without requiring deep technical knowledge.
6. Partnering with Specialised DI AI Providers: Often, the quickest and most effective way to bridge the gap between current capabilities and the potential offered by DI AI is to partner with specialized providers. These providers bring not only the technology but also the expertise in integrating and managing these systems, tailoring their tools to the specific needs of the business, and providing ongoing support to ensure the solutions evolve with the company’s needs.
Conclusion
CMOs embracing Decision Intelligence AI gain a profound competitive edge, maximising marketing ROI and driving tangible business growth. Don’t let complex data and disparate tools hinder your success. By partnering with the right DI AI solutions built around your existing systems, you’ll unlock unparalleled data-driven insights for exceptional marketing outcomes.
Are you ready for DI AI to revolutionise your marketing? Contact an expert today and elevate data into your most powerful strategic asset.
Want to Learn more?
Download the free e-book now : CFOs vs CMOs in Retail. 4 Practical Steps to Striking the Right Balance in Marketing Spend
You may also like: