In today’s digital age, data has become the lifeblood of modern businesses. However, simply collecting data is not enough; organizations must learn to effectively manage, analyse, and leverage it to make informed decisions and gain a competitive edge. This is where the concept of data maturity becomes crucial. Data maturity represents the evolution of how an organization handles and utilizes its data, moving from basic, unstructured data collection to advanced, AI-driven analytics.
The data maturity curve outlines the journey an organization takes, from having disorganized and inaccessible data to reaching a stage where data-driven insights are seamlessly integrated into decision-making processes. Understanding the stages of data maturity helps businesses recognize where they currently stand and what steps they need to take to improve their data capabilities.
By progressing along the data maturity curve, organizations can unlock increasingly sophisticated levels of data utilization, from descriptive analytics to prescriptive, AI-driven recommendations. Let’s explore the six key stages of this journey and how each step empowers businesses to achieve greater success.
The Six Stages of Data Maturity
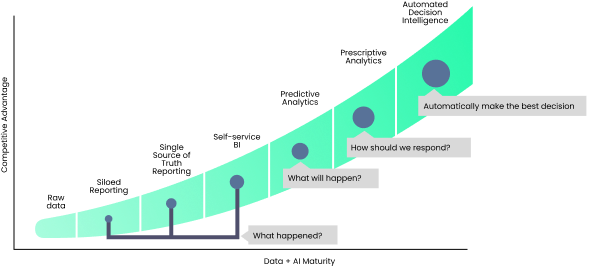
1. Siloed (Initial Stage)
At this initial stage, data is scattered across various sources within the organization. Departments operate in isolation, each maintaining its own set of data with little to no sharing between units. This fragmentation leads to several challenges:
1.Limited access to data across the organization
2.Inconsistent data formats and definitions
3.Difficulty in obtaining a holistic view of business operations
The primary focus at this stage is to begin centralizing data collection and storage. This initial step allows the organization to start accessing and using data that was previously dispersed and inaccessible. While analytics at this stage are basic and often ad-hoc, it marks the beginning of a data-driven journey.
Challenges:
- Lack of data visibility across departments
- Inconsistent data definitions and formats
- Difficulty in obtaining a comprehensive view of business operations
- Limited trust in data due to inconsistencies
Tips for Advancement:
- Conduct a data audit to identify all data sources within the organization
- Develop a centralization strategy, prioritizing critical data sets
- Begin standardizing data definitions across departments
- Invest in basic data integration tools
2. Centralized (Reactive Stage)
As organizations move to the second stage, they focus on centralizing their data. This crucial step involves:
1.Consolidating data sources into a central repository
2.Improving data accessibility across the organization
3.Initiating the definition and use of basic metrics
At this stage, leadership begins to introduce rudimentary self-service capabilities, allowing users to perform simple queries and analyses. While data quality issues may still persist, this centralization lays the foundation for more consistent decision-making and utilization of data.
The technology focus shifts to establishing a unified platform for data storage and initiating basic data cleaning processes. This stage represents a significant leap from siloed operations, enabling the organization to start breaking down data barriers between departments.
Challenges:
- Resistance to change from departments used to controlling their own data
- Initial data quality issues when combining disparate sources
- Need for new skills to manage centralized data systems
Tips for Advancement:
- Implement data governance policies to ensure data quality and consistency
- Provide training on new centralized data systems
- Start developing organization-wide KPIs
- Begin exploring data cleaning and standardization tools
3. Clean and Standardized (Defined Stage)
With data centralized, the next critical step is to ensure its quality and consistency. This stage involves:
1.Implementing robust data cleaning and standardization processes
2.Developing consistent definitions for metrics across the organization
3.Enhancing data reliability for more accurate analysis and decision-making
Leadership at this stage ensures that data cleaning and standardizing processes are refined, resulting in high-quality and consistent data. This increased data reliability builds trust among users, encouraging more data-driven decision-making throughout the organization.
From a technology perspective, this stage sees the enhancement of data quality management practices and the implementation of more sophisticated analytics models. The analytics team can now develop more advanced and standardized metrics, leading to improved strategic decision-making capabilities.
Challenges:
- Time and resource investment required for thorough data cleaning
- Balancing speed of data availability with quality assurance
- Maintaining consistent data standards across the organization
Tips for Advancement:
- Implement automated data quality checks and cleaning processes
- Develop a data dictionary for standardized definitions across the organization
- Establish a data stewardship program to maintain data quality
- Start connecting different data systems for a more integrated view
4. Connected (Managed Stage)
The fourth stage focuses on connecting various data sources and systems to integrate data across the organization. Key aspects include:
1.Seamless data flow between different systems and departments
2.Comprehensive coverage of business operations through connected data
3.Implementation of more sophisticated analytics, including predictive models
At this stage, leadership ensures that data connectivity is seamless, allowing for real-time data flow and comprehensive analysis. The analytics team now has access to a broader and more comprehensive dataset, enabling them to implement predictive and prescriptive analytics.
Technologically, this stage sees the optimization of data integration capabilities for real-time access and the deployment of advanced analytics models and AI algorithms for predictive insights.
Challenges:
- Ensuring data security and privacy across connected systems
- Managing real-time data integration and potential system conflicts
- Developing skills for more advanced analytics among team members
Tips for Advancement:
- Implement a robust data integration platform
- Provide training on predictive analytics and data modeling
- Start developing self-service analytics capabilities for basic use cases
- Begin exploring AI and machine learning applications
5. Self-Serve (Optimized Stage)
The fifth stage empowers users across the organization with self-service analytics capabilities. This involves:
1.Implementing intuitive, user-friendly analytics tools
2.Providing training to enhance data literacy across the organization
3.Enabling users to perform advanced analyses independently
Leadership at this stage focuses on expanding self-service capabilities, granting more users access to robust analytics tools for informed decision-making. This democratization of data leads to higher data literacy and embeds metrics and analytics into business processes.
From a technology standpoint, this stage sees the implementation of advanced self-service analytics platforms, often augmented with AI-driven insights to assist users in their analysis.
Challenges:
- Ensuring data literacy across a broad user base
- Balancing self-service capabilities with data governance
- Managing increased demand on data infrastructure
Tips for Advancement:
- Implement advanced self-service analytics tools with built-in governance
- Develop a comprehensive data literacy program
- Create centers of excellence to support advanced analytics use cases
- Begin integrating AI-driven insights into self-service tools
6. Prescriptive Analytics / AI (Leading Stage)
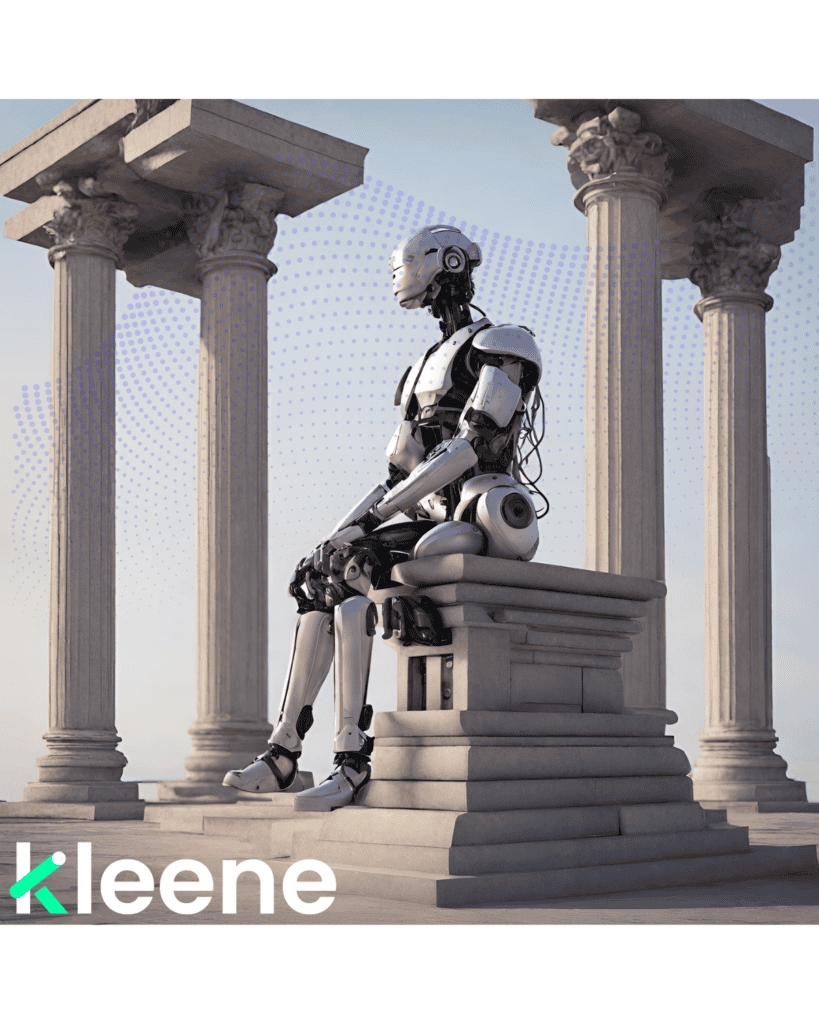
The final stage represents the pinnacle of data maturity, where organizations leverage cutting-edge AI and prescriptive analytics. Key features include:
1.Real-time, AI-driven decision-making processes
2.Continuous optimization and innovation through data
3.Integration of external and unstructured data sources
Leadership at this stage ensures that cutting-edge AI and prescriptive analytics capabilities are embedded into processes, continuously optimizing operations and driving innovation. The analytics team operates with a high degree of trust in the data, underpinned by a culture of data excellence and continuous improvement.
Technologically, this stage is characterized by the implementation of sophisticated AI models, prescriptive analytics solutions, and fully automated, intuitive self-service analytics tools.
Challenges:
- Keeping pace with rapidly evolving AI and analytics technologies
- Ensuring ethical use of AI in decision-making processes
- Maintaining human oversight and interpretation of AI-driven insights
Tips for Continuous Improvement:
- Establish an AI ethics committee to guide responsible AI use
- Continuously explore and test new AI and analytics technologies
- Foster a culture of innovation and continuous learning
- Develop partnerships with academic institutions or tech companies for cutting-edge insights
Conclusion
The data maturity curve represents more than just a technical evolution; it is a continuous journey that affects all aspects of an organization’s operations. As businesses progress through the stages of data maturity, they shift from reactive data use to proactive, AI-driven decision-making. This transformation not only boosts operational efficiency but also ignites innovation, giving organizations a competitive edge in today’s data-driven world.
No matter where your organization currently stands on the data maturity curve, it’s important to recognize the value of advancing along this path. Regularly assessing your level of data maturity and planning for future progression will ensure your business stays agile and competitive in an ever-changing environment.
Achieving data maturity isn’t just about implementing new technologies. It requires a balanced approach that includes leadership commitment, fostering a data-driven culture, and promoting continuous learning. By embracing this journey, your organization can unlock the full potential of its data and drive long-term success.
Want to learn more? there are three other ways you can get value from Kleene.ai:
- Download our “A Step-By-Step Guide to Getting From Raw Data to Decision Intelligence” eBook
- Watch our free on demand webinar with Bella & Duke, analysing their growth blueprint and how they optimised their LTV/CAC
- Book a call with an expert and learn how retailers are achieving automated decision intelligence https://kleene.ai/talk-to-an-expert/